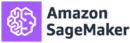
SageMaker
by Amazon | Founded 1994, Seattle, WA
Categories:
What is SageMaker?
Industry Specialties: Healthcare, finance, retail, manufacturing, energy, and more.
Amazon SageMaker is a comprehensive machine learning platform by Amazon Web Services (AWS) designed to simplify the entire machine learning lifecycle. It empowers businesses to build, train, deploy, and manage machine learning models efficiently. Key features include robust data preprocessing tools, a wide selection of machine learning algorithms, and automated hyperparameter tuning. SageMaker's scalability ensures it's suitable for both small experiments and large-scale production deployments. It offers cost-efficiency with a pay-as-you-go pricing model and facilitates model management and monitoring. The platform integrates seamlessly with the AWS ecosystem, providing security and compliance features. SageMaker's AutoML capabilities make machine learning accessible to users of varying expertise. Overall, it streamlines the machine learning process, enabling organizations to harness the power of AI for improved decision-making and innovation.
PRICE
$
$
$
$
$
COMPANY SIZE
DEPLOYMENT
PLATFORM
Try Before You Buy.
Request a Free Demo Today!
Request Demo
It's completely free!
SageMaker Pricing
Based on our most recent analysis, SageMaker pricing and cost details are described here:
- Price
- $$$$$
- Starting From
- $0.51
- Pricing Model
- Hourly
- Free Trial
- Request for Free
Training Resources
SageMaker is supported with the following types of training:
- Documentation
- In Person
- Live Online
- Videos
- Webinars
Support
The following support services are available for SageMaker:
- Phone
- Chat
- FAQ
- Forum
- Knowledge Base
- 24/7 Live Support
SageMaker Benefits and Insights
Why use SageMaker?
Key differentiators & advantages of SageMaker
- Accelerated Machine Learning: Amazon SageMaker offers a robust environment for building, training, and deploying machine learning models quickly and efficiently. It streamlines the ML workflow, reducing time-to-market.
- Scalability: With SageMaker, you can effortlessly scale your machine learning projects. It can handle both small-scale experiments and large-scale production deployments, ensuring flexibility as your needs evolve.
- Cost Efficiency: SageMaker's pay-as-you-go pricing model and built-in cost optimization tools help you manage expenses effectively. It optimizes resource allocation, preventing unnecessary spending.
- Managed Infrastructure: The service abstracts the complexities of infrastructure management. This allows data scientists and developers to focus on model development rather than worrying about provisioning and maintaining infrastructure.
- AutoML Capabilities: SageMaker provides AutoML features that automate aspects of model selection, hyperparameter tuning, and deployment, making it accessible to users with varying levels of expertise.
- Robust Data Labeling: SageMaker includes data labeling tools and integration with Amazon Mechanical Turk, making it easier to annotate and prepare data for training, a critical step in machine learning workflows.
- Secure and Compliant: Amazon SageMaker adheres to industry-leading security and compliance standards. It encrypts data, monitors access, and offers tools for compliance with regulations like GDPR and HIPAA.
- Customizable Workflows: SageMaker's flexibility allows you to customize your machine learning workflows to suit your specific requirements. You can integrate your own algorithms, libraries, and tools seamlessly.
- Model Management: It simplifies model management, versioning, and deployment, making it easy to keep track of different iterations of your models and roll out updates effortlessly.
- Real-time Inference: SageMaker supports real-time model inference, enabling you to integrate machine learning predictions into your applications and services in real-time, enhancing user experiences.
Industry Expertise
Amazon SageMaker boasts industry expertise in various sectors, including healthcare, financial services, retail, and manufacturing. Its specialized capabilities cater to the unique demands of each industry, such as healthcare's need for HIPAA compliance or retail's demand for recommendation systems. With SageMaker, businesses can harness tailored machine learning solutions to address specific challenges and opportunities within their respective sectors.
Synopsis of User Ratings and Reviews
Based on an aggregate of SageMaker reviews taken from the sources above, the following pros & cons have been curated by a SelectHub Market Analyst.
Pros
Cons
Researcher's Summary:
User reviews of Amazon SageMaker reveal a platform appreciated for its robust feature set, scalability, and cost-efficiency. Many users find its comprehensive tools for data preprocessing, model training, deployment, and monitoring to be a significant strength. Scalability is another key advantage, with SageMaker accommodating both small-scale experiments and large-scale production workloads effectively.
However, some users point out that SageMaker has a steep learning curve, particularly for beginners, and cost management can be challenging, especially during extensive model training. The platform's dependency on the broader AWS ecosystem can lead to vendor lock-in, which may not be ideal for organizations seeking flexibility.
SageMaker's AutoML capabilities, such as Autopilot, are praised for automating complex tasks, but some advanced users note limitations in customization. Additionally, while designed for real-time inference, it may not be optimized for batch processing or offline use cases.
In comparison to similar products, SageMaker stands out for its deep integration with AWS services, making it a preferred choice for those already within the AWS ecosystem. However, the learning curve and potential cost challenges are factors that users weigh against its benefits.
The platform's active community support and extensive documentation receive positive mentions, contributing to a smoother user experience. Overall, Amazon SageMaker is a powerful tool for machine learning but requires careful consideration of its complexities and potential cost implications.
Key Features
- Data Preprocessing Tools: SageMaker offers a range of data preprocessing capabilities, including data cleaning, transformation, and feature engineering, enabling users to prepare data efficiently for machine learning.
- Wide Model Selection: Users have access to a diverse library of machine learning algorithms, from linear regression to deep learning frameworks like TensorFlow, making it suitable for a variety of use cases.
- Hyperparameter Tuning: SageMaker automates hyperparameter optimization, helping users find the best configurations for their models, which can significantly improve model performance.
- Model Training at Scale: It supports distributed training across multiple instances, reducing training times and enabling the handling of large datasets with ease.
- Model Deployment: Users can deploy models as RESTful APIs, facilitating real-time inference in applications and services, and manage multiple model versions seamlessly.
- AutoML Capabilities: SageMaker Autopilot streamlines model creation for users without deep machine learning expertise, automating tasks like feature engineering and model selection.
- Monitoring and Debugging: It offers tools for model monitoring and debugging, helping users detect and address issues in deployed models, ensuring reliability in production.
- Explainability and Bias Detection: SageMaker provides features for model explainability and bias detection, essential for understanding model predictions and addressing ethical considerations.
- Integration with AWS Ecosystem: Seamlessly integrates with other AWS services, such as S3, Lambda, and Step Functions, facilitating end-to-end machine learning workflows within the AWS environment.
- Security and Compliance: Offers comprehensive security features, including data encryption, access control, and compliance with industry standards, making it suitable for sensitive industries like healthcare and finance.
- Cost Optimization: SageMaker includes cost optimization tools like automatic model scaling, enabling users to manage and optimize machine learning expenses efficiently.
Limitations
Some of the product limitations include:
- Learning Curve: SageMaker can have a steep learning curve, especially for those new to machine learning, due to its comprehensive set of features and tools.
- Cost Management: Users need to be vigilant about cost management, as complex machine learning workflows can lead to unexpected expenses.
- Customization Constraints: Advanced users may encounter limitations when customizing certain aspects of the SageMaker environment and algorithms.
- Data Privacy: SageMaker relies on cloud-based data storage, which may pose data privacy concerns for organizations with strict regulatory requirements.
- Dependency on AWS: To maximize its potential, SageMaker often requires integration with other AWS services, potentially leading to vendor lock-in.
- Offline Processing: While designed for real-time inference, SageMaker may not be optimized for batch processing or offline use cases.
- Resource Constraints: The platform's performance can be limited by the chosen instance types, affecting the speed of model training and inference.